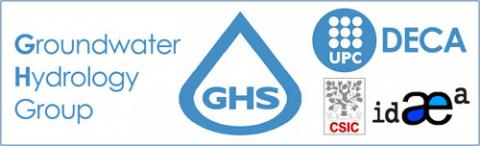
Webinar cycle in Hydrogeology and Geochemistry
HYDROGEOLOGY GROUP (Associated Unit CSIC-UPC, Barcelona)
Date: Thursday, 2nd November 2023
Starting time: 3:00 pm (Central European Time)
Author: Sara Elizabeth Hanson Hedgecock (IDAEA-CSIC), Postdoctoral Researcher
Title: Enhanced Geothermal Systems Reservoir and Induced Seismic Hazard Assessment Using Hybrid K-means Clustering and Data Fusion
Venue: UPC, D2 Module, 2nd floor classroom 216
Abstract:
Geothermal energy is a growing renewable resource that generates electricity and heat by circulating fluids in the subsurface.
Assessing reservoir quality and quantifying the amount of heat, fluid volume and circulation is key to determining a project’s economic viability. Where natural permeability is too low, high-pressure injection of fluids into the subsurface can be used to improve reservoir quality and increase the storage and production capacity of the system. These are called Enhanced Geothermal Systems (EGS).
Unfortunately, the injection of fluids at EGS sites has resulted in some felt induced seismic events (>Mw 2.5), such as in Soultz, France and Basel, Switzerland. Shaking from the events caused alarm in surrounding regions and shut down development of the projects.
Evaluating induced seismic hazard during EGS project planning is key to mitigating property damage and negative public perception that can shut down a site.
EGS site reservoir models are developed to fully characterize the geologic and geomechanical properties to aid treatment planning and to assess seismic hazards. These use a wide variety of data about previous seismic activity, geology, stress state, and poroelastic properties. Integrating the large variety of data is difficult due to the uncertainty and assumptions made in the data, processing, and modelling.
A probabilistic assessment system is developed that uses a combination of unsupervised hybrid k-means clustering and supervised learning to aid processing of multi-disciplinary data sets. Temperature, pressure, stress, porosity and permeability data from the FORGE EGS test site in Utah is used to build and test the system. This data is mapped in 3D and unsupervised clustering identifies initial clusters of patterns using the suite of input data. The initial data clusters are combined and assigned a final grouping using a pairwise combination method within the framework of the Transferable Belief Model, which is used to quantitatively represent the level of support and ignorance for combined cluster assignments.
Initial results classify the site into 3 to 5 clusters that correspond to variations in the input properties. The 3 clusters can be described as groups with (1) high (2) medium, and (3) low values of pressure, temperature, and stress magnitude. The run with 5 clusters divides the high medium and low clusters into 2 additional clusters. Cluster 2 and 5 have overlapping values, though the latter has a greater range of values.
The system developed in this work represents the first part of a planned system to aid the prediction of induced seismic events in EGS.
The second part of the planned system will use the identified property clusters in combination with fracture logs, treatment data, and microseismic events to identify likely regions of fracture growth.